|
|
[English](README.md) | 简体中文
|
|
|
# PaddleOCR Android Demo 使用文档
|
|
|
|
|
|
在 Android 上实现实时的PaddleOCR文字识别功能,此 Demo 有很好的的易用性和开放性,如在 Demo 中跑自己训练好的模型等。
|
|
|
|
|
|
## 环境准备
|
|
|
|
|
|
1. 在本地环境安装好 Android Studio 工具,详细安装方法请见[Android Stuido 官网](https://developer.android.com/studio)。
|
|
|
2. 准备一部 Android 手机,并开启 USB 调试模式。开启方法: `手机设置 -> 查找开发者选项 -> 打开开发者选项和 USB 调试模式`
|
|
|
|
|
|
## 部署步骤
|
|
|
|
|
|
1. 用 Android Studio 打开 PP-OCRv3/android 工程
|
|
|
2. 手机连接电脑,打开 USB 调试和文件传输模式,并在 Android Studio 上连接自己的手机设备(手机需要开启允许从 USB 安装软件权限)
|
|
|
|
|
|
<p align="center">
|
|
|
<img width="1440" alt="image" src="https://user-images.githubusercontent.com/31974251/203257262-71b908ab-bb2b-47d3-9efb-67631687b774.png">
|
|
|
</p>
|
|
|
|
|
|
> **注意:**
|
|
|
>> 如果您在导入项目、编译或者运行过程中遇到 NDK 配置错误的提示,请打开 ` File > Project Structure > SDK Location`,修改 `Andriod SDK location` 为您本机配置的 SDK 所在路径。
|
|
|
|
|
|
4. 点击 Run 按钮,自动编译 APP 并安装到手机。(该过程会自动下载预编译的 FastDeploy Android 库 以及 模型文件,需要联网)
|
|
|
成功后效果如下,图一:APP 安装到手机;图二: APP 打开后的效果,会自动识别图片中的物体并标记;图三:APP设置选项,点击右上角的设置图片,可以设置不同选项进行体验。
|
|
|
|
|
|
| APP 图标 | APP 效果 | APP设置项
|
|
|
| --- | --- | --- |
|
|
|
| 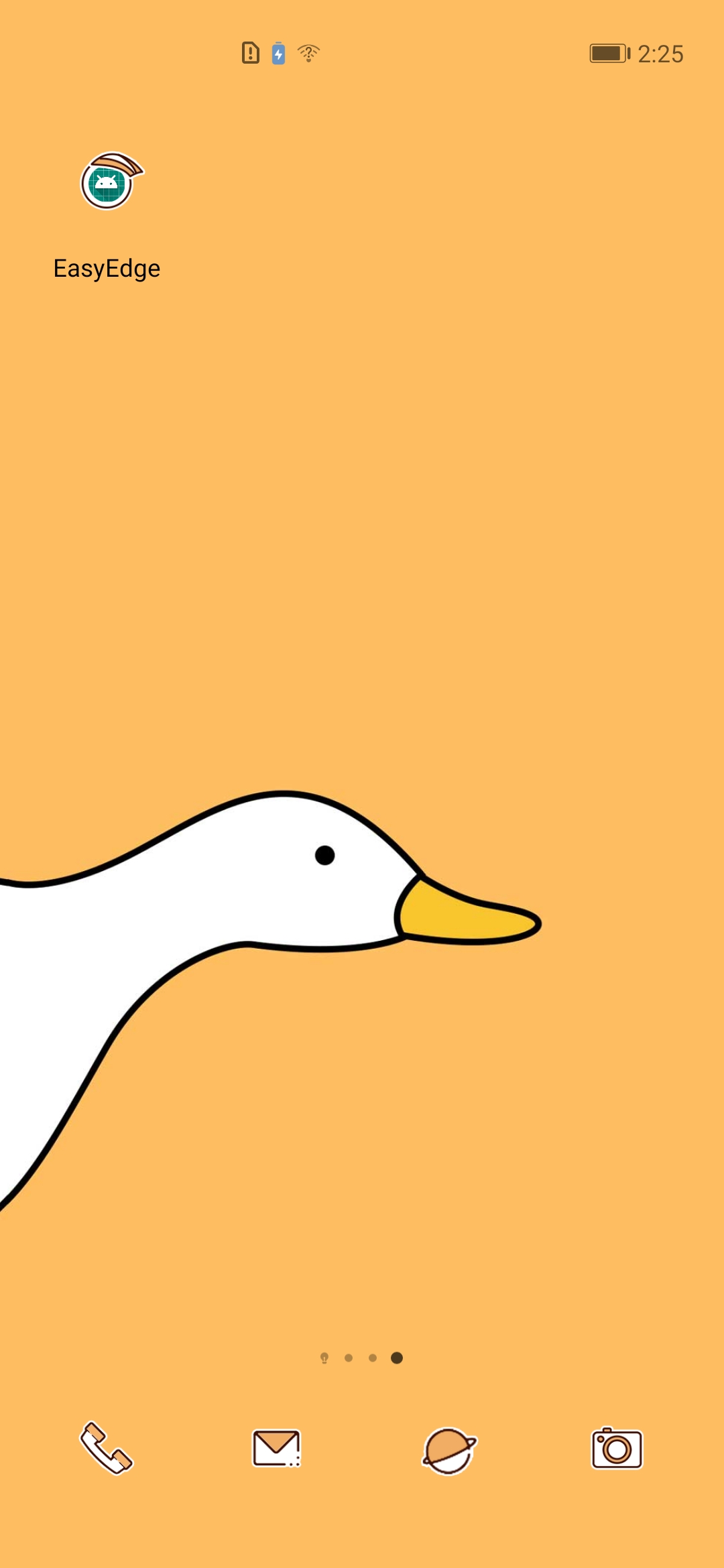 | 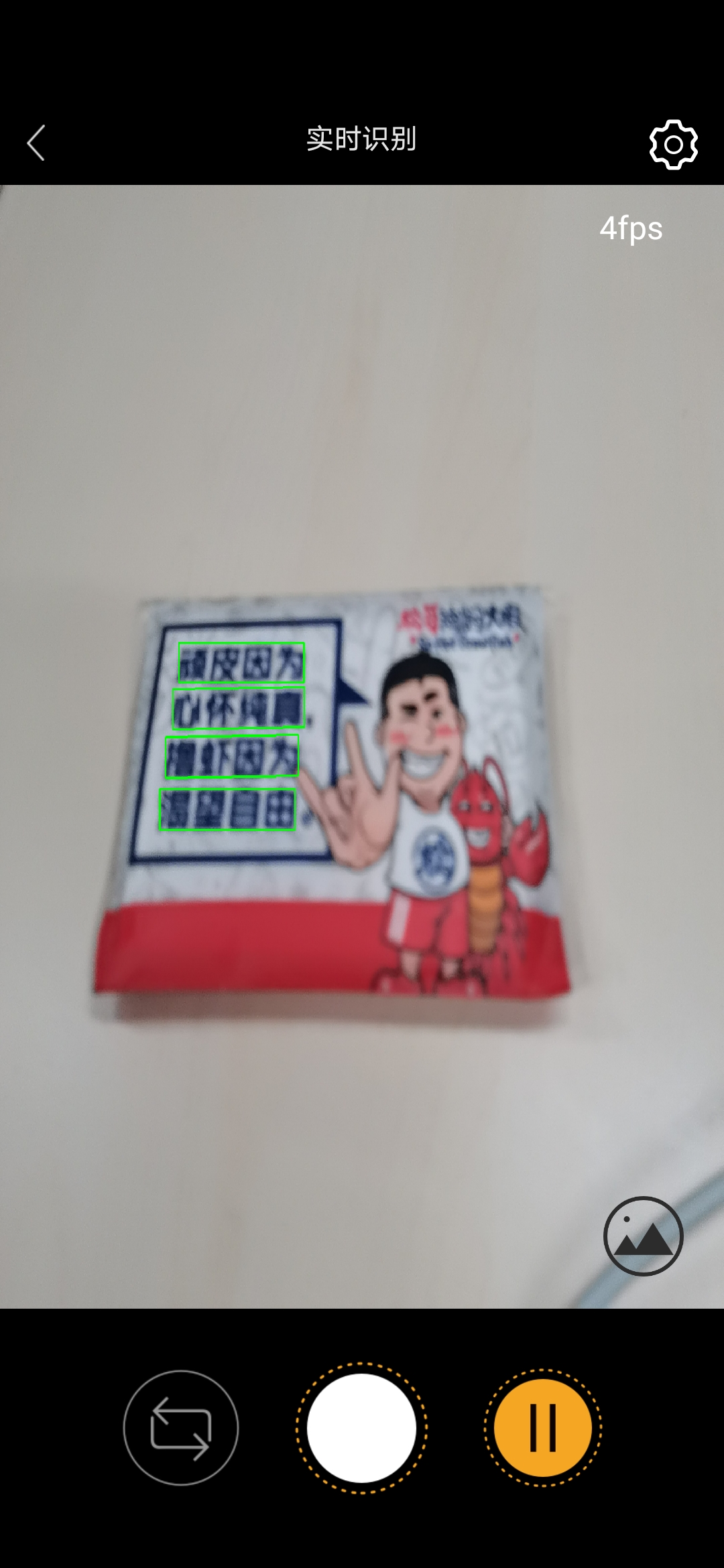 | 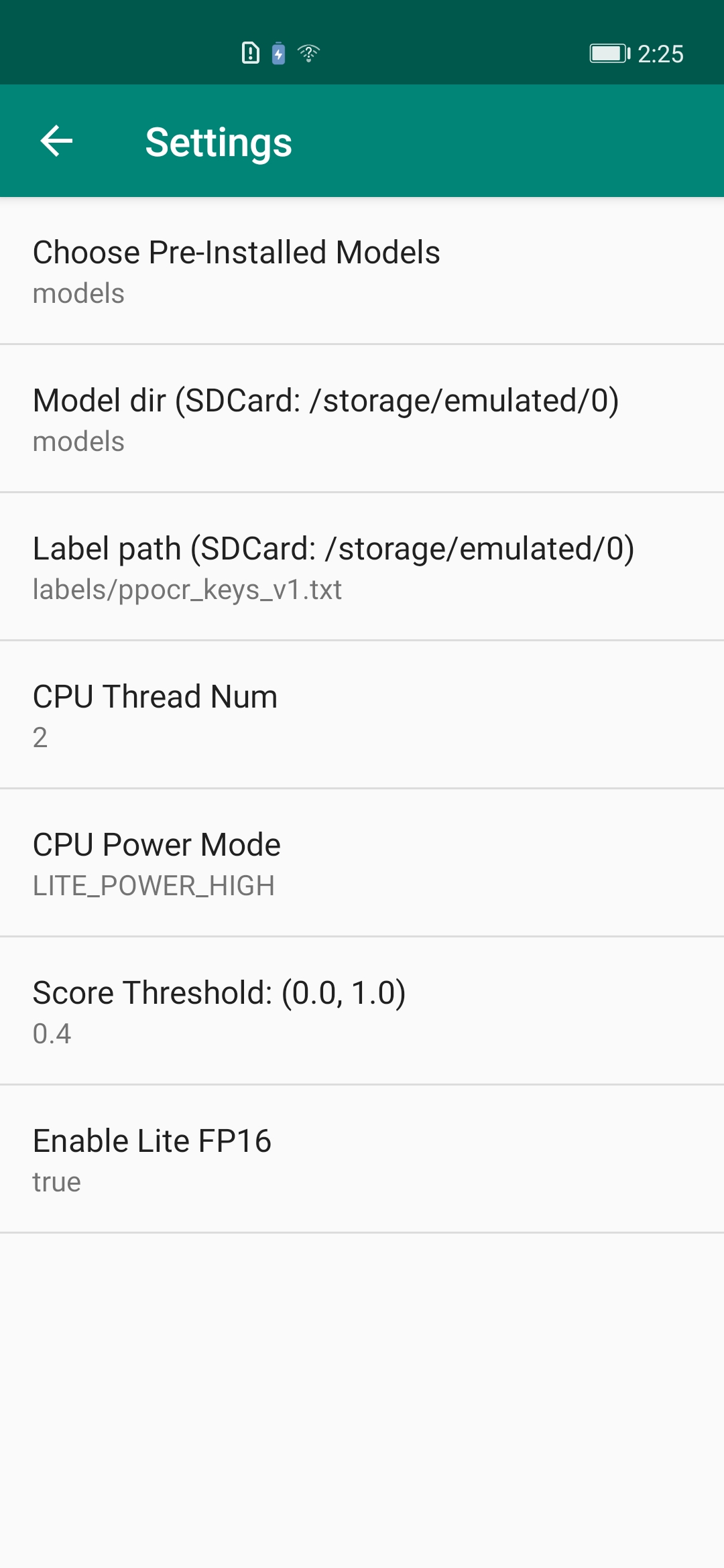 |
|
|
|
|
|
|
### PP-OCRv3 Java API 说明
|
|
|
|
|
|
- 模型初始化 API: 模型初始化API包含两种方式,方式一是通过构造函数直接初始化;方式二是,通过调用init函数,在合适的程序节点进行初始化。 PP-OCR初始化参数说明如下:
|
|
|
- modelFile: String, paddle格式的模型文件路径,如 model.pdmodel
|
|
|
- paramFile: String, paddle格式的参数文件路径,如 model.pdiparams
|
|
|
- labelFile: String, 可选参数,表示label标签文件所在路径,用于可视化,如 ppocr_keys_v1.txt,每一行包含一个label
|
|
|
- option: RuntimeOption,可选参数,模型初始化option。如果不传入该参数则会使用默认的运行时选项。
|
|
|
与其他模型不同的是,PP-OCRv3 包含 DBDetector、Classifier和Recognizer等基础模型,以及pipeline类型。
|
|
|
```java
|
|
|
// 构造函数: constructor w/o label file
|
|
|
public DBDetector(String modelFile, String paramsFile);
|
|
|
public DBDetector(String modelFile, String paramsFile, RuntimeOption option);
|
|
|
public Classifier(String modelFile, String paramsFile);
|
|
|
public Classifier(String modelFile, String paramsFile, RuntimeOption option);
|
|
|
public Recognizer(String modelFile, String paramsFile, String labelPath);
|
|
|
public Recognizer(String modelFile, String paramsFile, String labelPath, RuntimeOption option);
|
|
|
public PPOCRv3(); // 空构造函数,之后可以调用init初始化
|
|
|
// Constructor w/o classifier
|
|
|
public PPOCRv3(DBDetector detModel, Recognizer recModel);
|
|
|
public PPOCRv3(DBDetector detModel, Classifier clsModel, Recognizer recModel);
|
|
|
```
|
|
|
- 模型预测 API:模型预测API包含直接预测的API以及带可视化功能的API。直接预测是指,不保存图片以及不渲染结果到Bitmap上,仅预测推理结果。预测并且可视化是指,预测结果以及可视化,并将可视化后的图片保存到指定的途径,以及将可视化结果渲染在Bitmap(目前支持ARGB8888格式的Bitmap), 后续可将该Bitmap在camera中进行显示。
|
|
|
```java
|
|
|
// 直接预测:不保存图片以及不渲染结果到Bitmap上
|
|
|
public OCRResult predict(Bitmap ARGB8888Bitmap);
|
|
|
// 预测并且可视化:预测结果以及可视化,并将可视化后的图片保存到指定的途径,以及将可视化结果渲染在Bitmap上
|
|
|
public OCRResult predict(Bitmap ARGB8888Bitmap, String savedImagePath);
|
|
|
public OCRResult predict(Bitmap ARGB8888Bitmap, boolean rendering); // 只渲染 不保存图片
|
|
|
```
|
|
|
- 模型资源释放 API:调用 release() API 可以释放模型资源,返回true表示释放成功,false表示失败;调用 initialized() 可以判断模型是否初始化成功,true表示初始化成功,false表示失败。
|
|
|
```java
|
|
|
public boolean release(); // 释放native资源
|
|
|
public boolean initialized(); // 检查是否初始化成功
|
|
|
```
|
|
|
|
|
|
- RuntimeOption设置说明
|
|
|
|
|
|
```java
|
|
|
public void enableLiteFp16(); // 开启fp16精度推理
|
|
|
public void disableLiteFP16(); // 关闭fp16精度推理
|
|
|
public void enableLiteInt8(); // 开启int8精度推理,针对量化模型
|
|
|
public void disableLiteInt8(); // 关闭int8精度推理
|
|
|
public void setCpuThreadNum(int threadNum); // 设置线程数
|
|
|
public void setLitePowerMode(LitePowerMode mode); // 设置能耗模式
|
|
|
public void setLitePowerMode(String modeStr); // 通过字符串形式设置能耗模式
|
|
|
```
|
|
|
|
|
|
- 模型结果OCRResult说明
|
|
|
```java
|
|
|
public class OCRResult {
|
|
|
public int[][] mBoxes; // 表示单张图片检测出来的所有目标框坐标,每个框以8个int数值依次表示框的4个坐标点,顺序为左下,右下,右上,左上
|
|
|
public String[] mText; // 表示多个文本框内被识别出来的文本内容
|
|
|
public float[] mRecScores; // 表示文本框内识别出来的文本的置信度
|
|
|
public float[] mClsScores; // 表示文本框的分类结果的置信度
|
|
|
public int[] mClsLabels; // 表示文本框的方向分类类别
|
|
|
public boolean mInitialized = false; // 检测结果是否有效
|
|
|
}
|
|
|
```
|
|
|
其他参考:C++/Python对应的OCRResult说明: [api/vision_results/ocr_result.md](https://github.com/PaddlePaddle/FastDeploy/blob/develop/docs/api/vision_results/ocr_result.md)
|
|
|
|
|
|
|
|
|
- 模型调用示例1:使用构造函数
|
|
|
```java
|
|
|
import java.nio.ByteBuffer;
|
|
|
import android.graphics.Bitmap;
|
|
|
import android.opengl.GLES20;
|
|
|
|
|
|
import com.baidu.paddle.fastdeploy.RuntimeOption;
|
|
|
import com.baidu.paddle.fastdeploy.LitePowerMode;
|
|
|
import com.baidu.paddle.fastdeploy.vision.OCRResult;
|
|
|
import com.baidu.paddle.fastdeploy.vision.ocr.Classifier;
|
|
|
import com.baidu.paddle.fastdeploy.vision.ocr.DBDetector;
|
|
|
import com.baidu.paddle.fastdeploy.vision.ocr.Recognizer;
|
|
|
|
|
|
// 模型路径
|
|
|
String detModelFile = "ch_PP-OCRv3_det_infer/inference.pdmodel";
|
|
|
String detParamsFile = "ch_PP-OCRv3_det_infer/inference.pdiparams";
|
|
|
String clsModelFile = "ch_ppocr_mobile_v2.0_cls_infer/inference.pdmodel";
|
|
|
String clsParamsFile = "ch_ppocr_mobile_v2.0_cls_infer/inference.pdiparams";
|
|
|
String recModelFile = "ch_PP-OCRv3_rec_infer/inference.pdmodel";
|
|
|
String recParamsFile = "ch_PP-OCRv3_rec_infer/inference.pdiparams";
|
|
|
String recLabelFilePath = "labels/ppocr_keys_v1.txt";
|
|
|
// 设置RuntimeOption
|
|
|
RuntimeOption detOption = new RuntimeOption();
|
|
|
RuntimeOption clsOption = new RuntimeOption();
|
|
|
RuntimeOption recOption = new RuntimeOption();
|
|
|
detOption.setCpuThreadNum(2);
|
|
|
clsOption.setCpuThreadNum(2);
|
|
|
recOption.setCpuThreadNum(2);
|
|
|
detOption.setLitePowerMode(LitePowerMode.LITE_POWER_HIGH);
|
|
|
clsOption.setLitePowerMode(LitePowerMode.LITE_POWER_HIGH);
|
|
|
recOption.setLitePowerMode(LitePowerMode.LITE_POWER_HIGH);
|
|
|
detOption.enableLiteFp16();
|
|
|
clsOption.enableLiteFp16();
|
|
|
recOption.enableLiteFp16();
|
|
|
// 初始化模型
|
|
|
DBDetector detModel = new DBDetector(detModelFile, detParamsFile, detOption);
|
|
|
Classifier clsModel = new Classifier(clsModelFile, clsParamsFile, clsOption);
|
|
|
Recognizer recModel = new Recognizer(recModelFile, recParamsFile, recLabelFilePath, recOption);
|
|
|
PPOCRv3 model = new PPOCRv3(detModel,clsModel,recModel);
|
|
|
|
|
|
// 读取图片: 以下仅为读取Bitmap的伪代码
|
|
|
ByteBuffer pixelBuffer = ByteBuffer.allocate(width * height * 4);
|
|
|
GLES20.glReadPixels(0, 0, width, height, GLES20.GL_RGBA, GLES20.GL_UNSIGNED_BYTE, pixelBuffer);
|
|
|
Bitmap ARGB8888ImageBitmap = Bitmap.createBitmap(width, height, Bitmap.Config.ARGB_8888);
|
|
|
ARGB8888ImageBitmap.copyPixelsFromBuffer(pixelBuffer);
|
|
|
|
|
|
// 模型推理
|
|
|
OCRResult result = model.predict(ARGB8888ImageBitmap);
|
|
|
|
|
|
// 释放模型资源
|
|
|
model.release();
|
|
|
```
|
|
|
|
|
|
- 模型调用示例2: 在合适的程序节点,手动调用init
|
|
|
```java
|
|
|
// import 同上 ...
|
|
|
import com.baidu.paddle.fastdeploy.RuntimeOption;
|
|
|
import com.baidu.paddle.fastdeploy.LitePowerMode;
|
|
|
import com.baidu.paddle.fastdeploy.vision.OCRResult;
|
|
|
import com.baidu.paddle.fastdeploy.vision.ocr.Classifier;
|
|
|
import com.baidu.paddle.fastdeploy.vision.ocr.DBDetector;
|
|
|
import com.baidu.paddle.fastdeploy.vision.ocr.Recognizer;
|
|
|
// 新建空模型
|
|
|
PPOCRv3 model = new PPOCRv3();
|
|
|
// 模型路径
|
|
|
String detModelFile = "ch_PP-OCRv3_det_infer/inference.pdmodel";
|
|
|
String detParamsFile = "ch_PP-OCRv3_det_infer/inference.pdiparams";
|
|
|
String clsModelFile = "ch_ppocr_mobile_v2.0_cls_infer/inference.pdmodel";
|
|
|
String clsParamsFile = "ch_ppocr_mobile_v2.0_cls_infer/inference.pdiparams";
|
|
|
String recModelFile = "ch_PP-OCRv3_rec_infer/inference.pdmodel";
|
|
|
String recParamsFile = "ch_PP-OCRv3_rec_infer/inference.pdiparams";
|
|
|
String recLabelFilePath = "labels/ppocr_keys_v1.txt";
|
|
|
// 设置RuntimeOption
|
|
|
RuntimeOption detOption = new RuntimeOption();
|
|
|
RuntimeOption clsOption = new RuntimeOption();
|
|
|
RuntimeOption recOption = new RuntimeOption();
|
|
|
detOption.setCpuThreadNum(2);
|
|
|
clsOption.setCpuThreadNum(2);
|
|
|
recOption.setCpuThreadNum(2);
|
|
|
detOption.setLitePowerMode(LitePowerMode.LITE_POWER_HIGH);
|
|
|
clsOption.setLitePowerMode(LitePowerMode.LITE_POWER_HIGH);
|
|
|
recOption.setLitePowerMode(LitePowerMode.LITE_POWER_HIGH);
|
|
|
detOption.enableLiteFp16();
|
|
|
clsOption.enableLiteFp16();
|
|
|
recOption.enableLiteFp16();
|
|
|
// 使用init函数初始化
|
|
|
DBDetector detModel = new DBDetector(detModelFile, detParamsFile, detOption);
|
|
|
Classifier clsModel = new Classifier(clsModelFile, clsParamsFile, clsOption);
|
|
|
Recognizer recModel = new Recognizer(recModelFile, recParamsFile, recLabelFilePath, recOption);
|
|
|
model.init(detModel, clsModel, recModel);
|
|
|
// Bitmap读取、模型预测、资源释放 同上 ...
|
|
|
```
|
|
|
更详细的用法请参考 [OcrMainActivity](./app/src/main/java/com/baidu/paddle/fastdeploy/app/examples/ocr/OcrMainActivity.java)中的用法
|
|
|
|
|
|
## 替换 FastDeploy SDK和模型
|
|
|
替换FastDeploy预测库和模型的步骤非常简单。预测库所在的位置为 `app/libs/fastdeploy-android-sdk-xxx.aar`,其中 `xxx` 表示当前您使用的预测库版本号。模型所在的位置为,`app/src/main/assets/models`。
|
|
|
- 替换FastDeploy Android SDK: 下载或编译最新的FastDeploy Android SDK,解压缩后放在 `app/libs` 目录下;详细配置文档可参考:
|
|
|
- [在 Android 中使用 FastDeploy Java SDK](https://github.com/PaddlePaddle/FastDeploy/tree/develop/java/android)
|
|
|
|
|
|
- 替换OCR模型的步骤:
|
|
|
- 将您的OCR模型放在 `app/src/main/assets/models` 目录下;
|
|
|
- 修改 `app/src/main/res/values/strings.xml` 中模型路径的默认值,如:
|
|
|
```xml
|
|
|
<!-- 将这个路径修改成您的模型 -->
|
|
|
<string name="OCR_MODEL_DIR_DEFAULT">models</string>
|
|
|
<string name="OCR_LABEL_PATH_DEFAULT">labels/ppocr_keys_v1.txt</string>
|
|
|
```
|
|
|
## 使用量化模型
|
|
|
如果您使用的是量化格式的模型,只需要使用RuntimeOption的enableLiteInt8()接口设置Int8精度推理即可。
|
|
|
```java
|
|
|
String detModelFile = "ch_ppocrv3_plate_det_quant/inference.pdmodel";
|
|
|
String detParamsFile = "ch_ppocrv3_plate_det_quant/inference.pdiparams";
|
|
|
String recModelFile = "ch_ppocrv3_plate_rec_distillation_quant/inference.pdmodel";
|
|
|
String recParamsFile = "ch_ppocrv3_plate_rec_distillation_quant/inference.pdiparams";
|
|
|
String recLabelFilePath = "ppocr_keys_v1.txt"; // ppocr_keys_v1.txt
|
|
|
RuntimeOption detOption = new RuntimeOption();
|
|
|
RuntimeOption recOption = new RuntimeOption();
|
|
|
// 使用Int8精度进行推理
|
|
|
detOption.enableLiteInt8();
|
|
|
recOption.enableLiteInt8();
|
|
|
// 初始化PP-OCRv3 Pipeline
|
|
|
PPOCRv3 predictor = new PPOCRv3();
|
|
|
DBDetector detModel = new DBDetector(detModelFile, detParamsFile, detOption);
|
|
|
Recognizer recModel = new Recognizer(recModelFile, recParamsFile, recLabelFilePath, recOption);
|
|
|
predictor.init(detModel, recModel);
|
|
|
```
|
|
|
在App中使用,可以参考 [OcrMainActivity.java](./app/src/main/java/com/baidu/paddle/fastdeploy/app/examples/ocr/OcrMainActivity.java) 中的用法。
|
|
|
|
|
|
## 更多参考文档
|
|
|
如果您想知道更多的FastDeploy Java API文档以及如何通过JNI来接入FastDeploy C++ API感兴趣,可以参考以下内容:
|
|
|
- [在 Android 中使用 FastDeploy Java SDK](https://github.com/PaddlePaddle/FastDeploy/tree/develop/java/android)
|
|
|
- [在 Android 中使用 FastDeploy C++ SDK](https://github.com/PaddlePaddle/FastDeploy/blob/develop/docs/cn/faq/use_cpp_sdk_on_android.md)
|
|
|
- 如果用户想要调整前后处理超参数、单独使用文字检测识别模型、使用其他模型等,更多详细文档与说明请参考[PP-OCR系列在CPU/GPU上的部署](../../cpu-gpu/python/README.md)
|